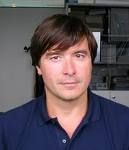
Cesare Furlanello
e-mail: furlan AT fbk.eu
website: mpba.fbk.eu
affiliation: Bruno Kessler Foundation (FBK), Trento
research area(s): Computational Biology
Course:
Biomolecular Sciences
University/Istitution: Università di Trento
University/Istitution: Università di Trento
Current position: Head of the Predictive Models for Biomedicine & Environment research unit (MPBA)
Center for Information Technology, Fondazione Bruno Kessler Via Sommarive 18,38123 Trento, Italy
Education and Professional Experience:
Nov 1986: "Laurea" in Mathematics, summa cum laude, University of Padua (Italy)
Jan 1987: Assistant research consultant, ITC-IRST, Trento
Apr 1988: tenured Researcher, ITC-IRST, Trento
1995-now: Head of Research Group, ITC-IRST
Sept 2000: Associate Senior Researcher, ITC-IRST, Trento
March 2008- : “1 fascia” Researcher, Bruno Kessler Foundation
1998-2001: Contract Professor “Applied Statistics for Biological Sciences”, Mathematics, Univ. Trento
2001-2003: Contract Professor “Computational Statistics and Predictive Models” , Univ. Trento
2004-2006: Statistical Machine Learning, Int. PhD School in ICT, Univ. Trento
2005: Spinoff company MPA Solutions launched
2001-2010: Founder and scientific director of the FBK summer school for interdisciplinary research
2010: Bioinformatics Collaborator, RIKEN Omics Center
Papers on international peer reviewed journals: 47; Book chapters: 5; Papers on national journals: 8; Int. congresses with review: 40. Total number of publications: 100
EU PROJECTS 2007-2011
FP7-EU Project HiPerDART (Development of High Performance Diagnostic Array Replication Technology), with Molecular Stamping, Royal Institute of Technology, University of Stockholm, Ecole Polytechnique Fédérale de Lausanne, Instituto Catalán de Oncologia, thinXXs Microtechnology AG, Crosslinks BV.
FP7-EU Project FluModCont (Modelling the spread of pandemic influenza and strategies for its containment and mitigation) with University of Trento, Imperial College London, Univ. Curie – Paris 6, Health Protection Agency UK, National Inst for Public Health and Environment NL, National Inst for Public Health and Welfare Finland, Univ. Bocconi Milano, Istituto Superiore di Sanità- National Centre for Epidemiology, Surveillance and Health Promotion IT, Public Health Institute Romania)
RESEARCH NETWORKS / NETWORKS OF EXCELLENCE
EuGESMA COST Action BM0801: Translating Genomic and Epigenetic Studies of MDS and AML (2010-2012)
FANTOM5 international consortium, RIKEN Omics Center (Yokohama) control mechanisms of functional variability of cells and difference of genetic networks between human individuals 2010-2012.
The MicroArray Quality Control (MAQC) Consortium, Food and Drug Administration (FDA) 2008-2011
Working Group “Machine learning for the environment”, National Center for Ecological Analysis and Synthesis – NCEAS center - NSF and Univ of California in S. Barbara (CA, USA), 2006-2008
Center for Information Technology, Fondazione Bruno Kessler Via Sommarive 18,38123 Trento, Italy
Education and Professional Experience:
Nov 1986: "Laurea" in Mathematics, summa cum laude, University of Padua (Italy)
Jan 1987: Assistant research consultant, ITC-IRST, Trento
Apr 1988: tenured Researcher, ITC-IRST, Trento
1995-now: Head of Research Group, ITC-IRST
Sept 2000: Associate Senior Researcher, ITC-IRST, Trento
March 2008- : “1 fascia” Researcher, Bruno Kessler Foundation
1998-2001: Contract Professor “Applied Statistics for Biological Sciences”, Mathematics, Univ. Trento
2001-2003: Contract Professor “Computational Statistics and Predictive Models” , Univ. Trento
2004-2006: Statistical Machine Learning, Int. PhD School in ICT, Univ. Trento
2005: Spinoff company MPA Solutions launched
2001-2010: Founder and scientific director of the FBK summer school for interdisciplinary research
2010: Bioinformatics Collaborator, RIKEN Omics Center
Papers on international peer reviewed journals: 47; Book chapters: 5; Papers on national journals: 8; Int. congresses with review: 40. Total number of publications: 100
EU PROJECTS 2007-2011
FP7-EU Project HiPerDART (Development of High Performance Diagnostic Array Replication Technology), with Molecular Stamping, Royal Institute of Technology, University of Stockholm, Ecole Polytechnique Fédérale de Lausanne, Instituto Catalán de Oncologia, thinXXs Microtechnology AG, Crosslinks BV.
FP7-EU Project FluModCont (Modelling the spread of pandemic influenza and strategies for its containment and mitigation) with University of Trento, Imperial College London, Univ. Curie – Paris 6, Health Protection Agency UK, National Inst for Public Health and Environment NL, National Inst for Public Health and Welfare Finland, Univ. Bocconi Milano, Istituto Superiore di Sanità- National Centre for Epidemiology, Surveillance and Health Promotion IT, Public Health Institute Romania)
RESEARCH NETWORKS / NETWORKS OF EXCELLENCE
EuGESMA COST Action BM0801: Translating Genomic and Epigenetic Studies of MDS and AML (2010-2012)
FANTOM5 international consortium, RIKEN Omics Center (Yokohama) control mechanisms of functional variability of cells and difference of genetic networks between human individuals 2010-2012.
The MicroArray Quality Control (MAQC) Consortium, Food and Drug Administration (FDA) 2008-2011
Working Group “Machine learning for the environment”, National Center for Ecological Analysis and Synthesis – NCEAS center - NSF and Univ of California in S. Barbara (CA, USA), 2006-2008
- Main research interest: applications of Machine Learning methods to genetic, medical and environmental data. Development of algorithms for classification and regression on high-throughput data, for model selection, for feature selection. Data visualization for decision support based on predictive models.
- Interdisciplinary research: basic and applied studies in life science and environmental risk (vector-borne disease mapping, wildlife epidemiology, traffic safety, landscape risk analysis, health indicators)
- Applications in Bioinformatics: since 2002, CF has contributed to the development of predictive classification models and gene selection procedures for molecular diagnostics, in collaboration with national and international centers of excellence in bioinformatics and molecular oncology.
- Development of IT methods for the integration of statistical machine learning methods in software infrastructures for data collection, management and distribution of predictive models, with more than 45 financed research projects for Environmental, Medical and geospatial risk analysis.
- Interdisciplinary research: basic and applied studies in life science and environmental risk (vector-borne disease mapping, wildlife epidemiology, traffic safety, landscape risk analysis, health indicators)
- Applications in Bioinformatics: since 2002, CF has contributed to the development of predictive classification models and gene selection procedures for molecular diagnostics, in collaboration with national and international centers of excellence in bioinformatics and molecular oncology.
- Development of IT methods for the integration of statistical machine learning methods in software infrastructures for data collection, management and distribution of predictive models, with more than 45 financed research projects for Environmental, Medical and geospatial risk analysis.
Li et al. The MAQC-II Project: A comprehensive study of common practices for the development and validation of microarray-based predictive models. Nature Biotechnology, 28(8):827-838, 2010.
Guzzetta, G., G. Jurman, C. Furlanello A machine learning pipeline for quantitative phenotype prediction from genotype data. BMC Bioinformatics, 11 Supp.8:S3, 2010.
K. Miclaus, M. Chierici, C. Lambert, L. Zhang, S. Vega, H. Hong, S. Yin, C. Furlanello, R. Wolfinger, F. Goodsaid. Variability in GWAS Analysis: the Impact of Genotype Calling Algorithm Inconsistencies. The Pharmacogenomics Journal, 10, 324-335, 2010.
G. Guzzetta, G. Jurman, C. Furlanello A machine learning for quantitative phenotype prediction from genotype data. BMC Bioinformatics, 11 Supp.8:S3, 2010
The MicroArray Quality Control (MAQC) Consortium. The MAQC-II Project: A comprehensive study of common practices for the development and validation of microarray-based predictive models. Nature Biotechnology, 28(8):827-838, 2010.
M. Chierici, K. Miclaus, S. Vega, C. Furlanello. An interactive effect of batch size and composition contributes to discordant results in GWAS with the CHIAMO genotyping algorithm. Pharmacogenomics J, 10, 355–363, 2010.
H. Hong, L. Shi, Z. Su, W. Ge, W. Jones, W. Czika, K. Miclaus, C. Lambert, S. Vega, J. Zhang, B. Ning, J. Liu, B. Green, L. Xu, H. Fang, R. Perkins, S. Lin, N. Jafari, K. Park, T. Ahn, M. Chierici, C. Furlanello, L. Zhang, R. Wolfinger, F. Goodsaid, W. Tong. Assessing sources of inconsistencies in genotypes and their effects on genome-wide association studies with HapMap samples. Pharmacogenomics J, 10, 364–374, 2010
L. Zhang, S. Yin, K. Miclaus, M. Chierici, S. Vega, C. Lambert, H. Hong, R. Wolfinger, C. Furlanello, F. Goodsaid. Assessment of variability in GWAS with CRLMM genotyping algorithm on WTCCC Coronary Artery Disease. Pharmacogenomics J, 10, 347–354, 2010
W. Shi, M. Bessarabova, D. Dosymbekov, Z. Dezso, T. Nikolskaya, M. Dudoladova, T. Serebryiskaya, A. Bugrim, R.J. Brennan, R. Shah, J. Dopazo, M. Chen, Y. Deng, T. Shi, G. Jurman, C. Furlanello, R.S. Thomas, J.C. Corton, W. Tong, L. Shi, Y, Nikolsky. Functional analysis reveals the biological underpinnings of predictive genomic signatures. Pharmacogenomics J , 10, 310–323, 2010.
K. Miclaus, M. Chierici, C. Lambert, L. Zhang, S. Vega, H. Hong, S. Yin, C. Furlanello, R. Wolfinger, F. Goodsaid. Variability in GWAS Analysis: the Impact of Genotype Calling Algorithm Inconsistencies. Pharmacogenomics J, 10, 324–335, 2010.
K. Miclaus, R. Wolfinger, S. Vega, M. Chierici, C. Furlanello, C. Lambert, H. Hong, L. Zhang, S. Yin, F. Goodsaid. Batch Effects in the BRLMM Genotype Calling Algorithm Influence GWAS Results for the Affymetrix 500K Array. Pharmacogenomics J, 10, 336–346, 2010.
Ioannidis, J.P.A., Allison, D.B., C.A. Ball, I. Coulibaly, X. Cui, A.C. Culhane, M. Falchi, C. Furlanello, L. Game, G. Jurman, T. Mehta, J. Mangion, M. Nitzberg, G.P. Page, E. Petretto, V. van Noort. Repeatability of published microarray gene expression analyses. Nature Genetics, 41(2):499-505, 2009.
M. Ciofi degli Atti, S. Merler, C. Rizzo, M. Ajelli, M. Massari, P. Manfredi, C. Furlanello, G. Scalia Tomba, and M. Iannelli. Mitigation measures for pandemic influenza in Italy: an individual based model considering different scenarios. PLoS ONE, 3(3): e1790, 2008.
A. Barla, G. Jurman, S. Riccadonna, M. Chierici, S. Merler, and C. Furlanello. Machine learning methods for predictive proteomics. Briefings in Bioinformatics, 9(2), 119-128, 2008
G. Jurman, S. Merler, A. Barla, S. Paoli, A. Galea, and C. Furlanello. Algebraic stability indicators for ranked lists in molecular profiling.. Bioinformatics, 24(2):258-264, 2008.
S.Paoli, G. Jurman, D. Albanese, S. Merler and C. Furlanello Integrating gene expression profiling and clinical data Int. J. of Approx. Reasoning, 47(1):58-69, 2008.
S. Riccadonna, G. Jurman, S. Merler, S. Paoli, A. Quattrone, and C. Furlanello. Supervised classification of combined copy number and gene expression data. Journal of Integrative Bioinformatics , 4(3):74:177-188, 2007.
M. Cannataro, A. Barla, R. Flor, A. Gallo, G. Jurman, S. Merler, S. Paoli, G. Tradigo, P. Veltri, and C. Furlanello A grid environment for high-throughput proteomics, IEEE Trans. on Nanobiosciences, 6(2):117-123, 2007.
M. L. Ciofi degli Atti, C. Rizzo, A. Bella, M. Massari, M. Iannelli, A. Lunelli, A. Pugliese, J. Ripoll, P. Manfredi, G. Scalia Tomba, S. Merler, G. Jurman, and C. Furlanello. Modelling scenarios of diffusion and control of pandemic influenza, Italy. Euro Surveill., 12(1): 2007
P. M. Granitto, F. Gasperi, F. Biasioli, E. Trainotti & C. Furlanello, "Modern data mining tools in descriptive sensory analysis: a case study with a Random Forest approach", Food Quality and Preferences , 18(4):681-689, 2007
Guzzetta, G., G. Jurman, C. Furlanello A machine learning pipeline for quantitative phenotype prediction from genotype data. BMC Bioinformatics, 11 Supp.8:S3, 2010.
K. Miclaus, M. Chierici, C. Lambert, L. Zhang, S. Vega, H. Hong, S. Yin, C. Furlanello, R. Wolfinger, F. Goodsaid. Variability in GWAS Analysis: the Impact of Genotype Calling Algorithm Inconsistencies. The Pharmacogenomics Journal, 10, 324-335, 2010.
G. Guzzetta, G. Jurman, C. Furlanello A machine learning for quantitative phenotype prediction from genotype data. BMC Bioinformatics, 11 Supp.8:S3, 2010
The MicroArray Quality Control (MAQC) Consortium. The MAQC-II Project: A comprehensive study of common practices for the development and validation of microarray-based predictive models. Nature Biotechnology, 28(8):827-838, 2010.
M. Chierici, K. Miclaus, S. Vega, C. Furlanello. An interactive effect of batch size and composition contributes to discordant results in GWAS with the CHIAMO genotyping algorithm. Pharmacogenomics J, 10, 355–363, 2010.
H. Hong, L. Shi, Z. Su, W. Ge, W. Jones, W. Czika, K. Miclaus, C. Lambert, S. Vega, J. Zhang, B. Ning, J. Liu, B. Green, L. Xu, H. Fang, R. Perkins, S. Lin, N. Jafari, K. Park, T. Ahn, M. Chierici, C. Furlanello, L. Zhang, R. Wolfinger, F. Goodsaid, W. Tong. Assessing sources of inconsistencies in genotypes and their effects on genome-wide association studies with HapMap samples. Pharmacogenomics J, 10, 364–374, 2010
L. Zhang, S. Yin, K. Miclaus, M. Chierici, S. Vega, C. Lambert, H. Hong, R. Wolfinger, C. Furlanello, F. Goodsaid. Assessment of variability in GWAS with CRLMM genotyping algorithm on WTCCC Coronary Artery Disease. Pharmacogenomics J, 10, 347–354, 2010
W. Shi, M. Bessarabova, D. Dosymbekov, Z. Dezso, T. Nikolskaya, M. Dudoladova, T. Serebryiskaya, A. Bugrim, R.J. Brennan, R. Shah, J. Dopazo, M. Chen, Y. Deng, T. Shi, G. Jurman, C. Furlanello, R.S. Thomas, J.C. Corton, W. Tong, L. Shi, Y, Nikolsky. Functional analysis reveals the biological underpinnings of predictive genomic signatures. Pharmacogenomics J , 10, 310–323, 2010.
K. Miclaus, M. Chierici, C. Lambert, L. Zhang, S. Vega, H. Hong, S. Yin, C. Furlanello, R. Wolfinger, F. Goodsaid. Variability in GWAS Analysis: the Impact of Genotype Calling Algorithm Inconsistencies. Pharmacogenomics J, 10, 324–335, 2010.
K. Miclaus, R. Wolfinger, S. Vega, M. Chierici, C. Furlanello, C. Lambert, H. Hong, L. Zhang, S. Yin, F. Goodsaid. Batch Effects in the BRLMM Genotype Calling Algorithm Influence GWAS Results for the Affymetrix 500K Array. Pharmacogenomics J, 10, 336–346, 2010.
Ioannidis, J.P.A., Allison, D.B., C.A. Ball, I. Coulibaly, X. Cui, A.C. Culhane, M. Falchi, C. Furlanello, L. Game, G. Jurman, T. Mehta, J. Mangion, M. Nitzberg, G.P. Page, E. Petretto, V. van Noort. Repeatability of published microarray gene expression analyses. Nature Genetics, 41(2):499-505, 2009.
M. Ciofi degli Atti, S. Merler, C. Rizzo, M. Ajelli, M. Massari, P. Manfredi, C. Furlanello, G. Scalia Tomba, and M. Iannelli. Mitigation measures for pandemic influenza in Italy: an individual based model considering different scenarios. PLoS ONE, 3(3): e1790, 2008.
A. Barla, G. Jurman, S. Riccadonna, M. Chierici, S. Merler, and C. Furlanello. Machine learning methods for predictive proteomics. Briefings in Bioinformatics, 9(2), 119-128, 2008
G. Jurman, S. Merler, A. Barla, S. Paoli, A. Galea, and C. Furlanello. Algebraic stability indicators for ranked lists in molecular profiling.. Bioinformatics, 24(2):258-264, 2008.
S.Paoli, G. Jurman, D. Albanese, S. Merler and C. Furlanello Integrating gene expression profiling and clinical data Int. J. of Approx. Reasoning, 47(1):58-69, 2008.
S. Riccadonna, G. Jurman, S. Merler, S. Paoli, A. Quattrone, and C. Furlanello. Supervised classification of combined copy number and gene expression data. Journal of Integrative Bioinformatics , 4(3):74:177-188, 2007.
M. Cannataro, A. Barla, R. Flor, A. Gallo, G. Jurman, S. Merler, S. Paoli, G. Tradigo, P. Veltri, and C. Furlanello A grid environment for high-throughput proteomics, IEEE Trans. on Nanobiosciences, 6(2):117-123, 2007.
M. L. Ciofi degli Atti, C. Rizzo, A. Bella, M. Massari, M. Iannelli, A. Lunelli, A. Pugliese, J. Ripoll, P. Manfredi, G. Scalia Tomba, S. Merler, G. Jurman, and C. Furlanello. Modelling scenarios of diffusion and control of pandemic influenza, Italy. Euro Surveill., 12(1): 2007
P. M. Granitto, F. Gasperi, F. Biasioli, E. Trainotti & C. Furlanello, "Modern data mining tools in descriptive sensory analysis: a case study with a Random Forest approach", Food Quality and Preferences , 18(4):681-689, 2007
Project Title:
Computational Functional Genomics
The ideal candidate will have a strong interest in developing an interdisciplinary expertise in computational methods for high-throughput functional genomics, with special attention to next generation sequencing. Some basic knowledge of the mechanisms of regulation at DNA, RNA (miRNA), and protein levels and of standard DNA/RNA sequencing concepts is required. Intermediate or higher IT skills are expected in algorithmics for bioinformatics, programming languages (Python and C as baseline), competence in the use of *nix O.S., in interacting with databases, use of standard bioinformatics tools, knowledge of the R statistical and visualization environment. Basic mathematics competence should cover calculus linear algebra. Bases of statistical machine learning are also required.