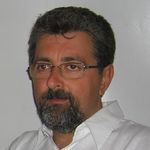
Gennaro Miele
e-mail: miele AT na.infn.it
affiliation: Università di Napoli Federico II
research area(s): Computational Biology
Course:
Computational Biology and Bioinformatics
University/Istitution: Università di Napoli Federico II
University/Istitution: Università di Napoli Federico II
Higher Education
July 1986 - Laurea in Fisica at the University of Naples "Federico II" with the maximum grade (110/110 cum laude), with a thesis entitled: Super-String inspired phenomenological models.
October 1990 - Ph.D. in Physics at the University of Naples "Federico II", with a thesis entitled: Nonleptonic decays of charmed and beauty pseudoscalar mesons.
Professional Affiliations
February 1990 - July 1991 - Post-Doctoral position of INFN.
July 1991 - October 2001 - Assistant Professor (Ricercatore) at the University of Naples "Federico II".
November 2001 " to present - Associate Professor of Theoretical Physics at the University of Naples "Federico II"
July 1986 - Laurea in Fisica at the University of Naples "Federico II" with the maximum grade (110/110 cum laude), with a thesis entitled: Super-String inspired phenomenological models.
October 1990 - Ph.D. in Physics at the University of Naples "Federico II", with a thesis entitled: Nonleptonic decays of charmed and beauty pseudoscalar mesons.
Professional Affiliations
February 1990 - July 1991 - Post-Doctoral position of INFN.
July 1991 - October 2001 - Assistant Professor (Ricercatore) at the University of Naples "Federico II".
November 2001 " to present - Associate Professor of Theoretical Physics at the University of Naples "Federico II"
Since 1986, when I got my first degree, I have worked in several fields of theoretical physics and more recently of bioinformatics/computational biology.
In Astroparticle Physics and Cosmology (in a random order) " still active in this sector:
Inflationary Models
NCG effects in cosmology
Big Bang Nucleosynthesis
Cosmological and astrophysical neutrinos
High energy neutrinos
Dark Matter
In Bioinformatics and Computational Biology (in random order) " a recently opened sector
Gene-environment interaction in modelling risk factor in complex diseases
Pattern recognition methods in bio-medical data analysis
Evolutionary genomics and the candidate gene selection
In Astroparticle Physics and Cosmology (in a random order) " still active in this sector:
Inflationary Models
NCG effects in cosmology
Big Bang Nucleosynthesis
Cosmological and astrophysical neutrinos
High energy neutrinos
Dark Matter
In Bioinformatics and Computational Biology (in random order) " a recently opened sector
Gene-environment interaction in modelling risk factor in complex diseases
Pattern recognition methods in bio-medical data analysis
Evolutionary genomics and the candidate gene selection
1) R. Amato, M. Pinelli, A. Monticelli, G. Miele, S. Cocozza SCHIZOPHRENIA AND VITAMIN D RELATED GENES COULD HAVE BEEN SUBJECT TO LATITUDE-DRIVEN ADAPTATIONBMC Evolutionary Biology, vol. 10 (2010); p.351,
2) R. Amato, M. Pinelli, D. D'andrea, G. Miele, M. Nicodemi, G. Raiconi, S. Cocozza A NOVEL APPROACH TO SIMULATE GENE-ENVIRONMENT INTERACTIONS IN COMPLEX DISEASES.BMC Bioinformatics, vol. 11 (2010) ; p. 8-1-8-18, ISSN: 1471-2105, doi:10.1186/1471-2105-11-8
3) R. Amato, M. Pinelli, A. Monticelli, D. Marino, G. Miele, S. Cocozza GENOME-WIDE SCAN FOR SIGNATURES OF HUMAN POPULATION DIFFERENTIATION AND THEIR RELATIONSHIP WITH NATURAL SELECTION, FUNCTIONAL PATHWAYS AND DISEASES.PLOS ONE, vol. 4 (2009); p.1-8, ISSN: 1932-6203,doi:10.1371/journal.pone.0007927
4) F. Verginelli, F. Bishehsari, F. Napolitano, M. Mahdavinia, A. Cama, R. Malekzadeh,G. Miele, G. Raiconi, R. Tagliaferri, R. Mariani-Costantini TRANSITIONS AT CPG DINUCLEOTIDES, GEOGRAPHIC CLUSTERING OF TP53 MUTATIONS AND FOOD AVAILABILITY PATTERNS IN COLORECTAL CANCER.PLOS ONE, vol. 4 (2009); p. e6824-1-e6824-15, ISSN: 1932-6203, doi:10.1371/journal.pone.0006824
5) A. Ciaramella, S. Cocozza, F. Iorio, G. Miele, F. Napolitano, M. Pinelli, G. Raiconi, R. Tagliaferri. INTERACTIVE DATA ANALYSIS AND CLUSTERING OF GENOMIC DATA Neural Networks 21,368-378, 2008
6) F. Napolitano, G. Raiconi, R. Tagliaferri, A. Ciaramella, G. Miele, A. Staiano CLUSTERING AND VISUALIZATION APPROACHES FOR HUMAN CELL CYCLE GENE EXPRESSION DATA ANALYSIS.
International Journal of Approximate Reasoning 47, 70-84, 2008
7) F. Bishehsari, M. Mahdavinia, R. Malekzadeh, R. Mariani-Costantini, G. Miele, F. Napolitano, G. Raiconi, R. Tagliaferri, F. Verginelli PCA BASED FEATURE SELECTION APPLIED TO THE ANALYSIS OF THE INTERNATIONAL VARIATION IN DIET. Lecture Notes in Artificial Intelligence 4578, 551-556, 2007
8) R. Tagliaferri, A. Ciaramella, S. Cocozza, F. Iorio, F. Napolitano, M. Pinelli, G. Raiconi and G. Miele. CLUSTERING, ASSESSMENT AND VALIDATION: AN APPLICATION TO GENE EXPRESSION DATA.International Joint Conference on Neural Networks (IJCNN 2007) : 1613-1518
9) R. Amato, A. Ciaramella, N. Deniskina, C. Del Mondo, D. di Bernardo, C. Donalek, G. Longo, G. Mangano, G. Miele, G. Raiconi, A. Staiano, R. Tagliaferri,NEC FOR GENE EXPRESSION ANALYSIS Fuzzy Logic And Applications Lecture Notes In Artificial Intelligence 3849 (2006) 246-251.
10) R. Amato, A. Ciaramella, N. Deniskina, C. Del Mondo, D. di Bernardo, C. Donalek, G. Longo, G. Mangano, G. Miele, G. Raiconi, A. Staiano, and R. Tagliaferri, A MULTI-STEP APPROACH TO TIME SERIES ANALYSIS AND GENE EXPRESSION CLUSTERING. Bioinformatics, 1 March 2006; 22: 589 - 596.
Project Title:
Timing of allelic variants under selective pressure involved in complex diseases
Background
New high throughput technologies, next- generation sequencing above all, are providing an increasing amount of data that are having a tremendous impact in several biological areas. In particular, in the population genetics field, many questions, unsolved for decades, potentially could find an answer.
Concerning the role of natural selection in featuring a given phenotype, several methods able to identify signature of selective pressure are available. Unfortunately, the age of onset of the selective pressure is hard to be estimated. This estimation could be useful to suggest hypotheses on the selective forces involved and, therefore, on “why” the selective event occurred.
A difficulty could arise from the occurrence of two different scenario. In the first one, the mutation undergoes to selective pressure at its appearance. In the second one, the selection acts on a standing variation
Among the most used approaches for allele age estimation, particular attention deserves the haplotype decay method. A relatively simple and widely used implementation of this method was proposed by Voight and colleagues (Voight, B. et al., 2006). Recombination distance decay exponentially with the time. In particular, given r the recombination distance at which the two chromosomes are still identical and T the tMRCA expressed in number of generations: P(Homo) = e-r T .This method makes some simplifying assumptions about the demographic model that make it unsuitable in many real applications. In particular, they assume a population that is panmitic and constant in size, which is clearly unrealistic for human.
Another interesting approach, known as counting method, comes from Thomson and colleagues (Thomson, R, et al. 2000). This method starts building a tree for the region of interest and counting the number of mutations. Since neutral mutations accumulate at a constant rate, the total number results to be
proportional to the tMRCA. Given xi the number of mutations on branch i (from the root to the tip, and thus the number of mutations carried by the i-th chromosome, n the number of chromosomes and μ the mutation rate, T= Σi xi/nμ .Conversely from the haplotype decay, this method makes no assumptions on the demographic model. However, it only considers one tree per region and thus its interpretation in presence of recombination is not straightforward.
Moreover, both these methods as well as many others, usually just provide a punctual estimation of the age and no general rules exist to assess the confidence interval of these estimations.
Very recently (Amato, R. PhD Thesis, 2011) the Approximate Bayesian Computation (ABC) method has been applied for the age determination of selective sweeps. The approach overcomes the problem of the knowledge of the exact likelihood calculation by means of simulations and a set of quantities, called “summary statistics” (SSs) that summarize the data.
Aim
The project of PhD thesis is aimed to a general improvement of such a method and to a wider application of it. In particular one would like to:
1) Extend to a genome wide scale the study, in particular with regard to genes involved in complex diseases in regions under selective pressure.
2) Improve the methods both in terms of computational efficiency and of quality of the estimations;
3) Generalize the applicability of the method to a wider range of scenarios (selection on standing variations, variants undergone selection but currently fixed, more complex demographic scenarios, regions with different recombination rates, and impact of epigenetic modifications, etc.);
New high throughput technologies, next- generation sequencing above all, are providing an increasing amount of data that are having a tremendous impact in several biological areas. In particular, in the population genetics field, many questions, unsolved for decades, potentially could find an answer.
Concerning the role of natural selection in featuring a given phenotype, several methods able to identify signature of selective pressure are available. Unfortunately, the age of onset of the selective pressure is hard to be estimated. This estimation could be useful to suggest hypotheses on the selective forces involved and, therefore, on “why” the selective event occurred.
A difficulty could arise from the occurrence of two different scenario. In the first one, the mutation undergoes to selective pressure at its appearance. In the second one, the selection acts on a standing variation
Among the most used approaches for allele age estimation, particular attention deserves the haplotype decay method. A relatively simple and widely used implementation of this method was proposed by Voight and colleagues (Voight, B. et al., 2006). Recombination distance decay exponentially with the time. In particular, given r the recombination distance at which the two chromosomes are still identical and T the tMRCA expressed in number of generations: P(Homo) = e-r T .This method makes some simplifying assumptions about the demographic model that make it unsuitable in many real applications. In particular, they assume a population that is panmitic and constant in size, which is clearly unrealistic for human.
Another interesting approach, known as counting method, comes from Thomson and colleagues (Thomson, R, et al. 2000). This method starts building a tree for the region of interest and counting the number of mutations. Since neutral mutations accumulate at a constant rate, the total number results to be
proportional to the tMRCA. Given xi the number of mutations on branch i (from the root to the tip, and thus the number of mutations carried by the i-th chromosome, n the number of chromosomes and μ the mutation rate, T= Σi xi/nμ .Conversely from the haplotype decay, this method makes no assumptions on the demographic model. However, it only considers one tree per region and thus its interpretation in presence of recombination is not straightforward.
Moreover, both these methods as well as many others, usually just provide a punctual estimation of the age and no general rules exist to assess the confidence interval of these estimations.
Very recently (Amato, R. PhD Thesis, 2011) the Approximate Bayesian Computation (ABC) method has been applied for the age determination of selective sweeps. The approach overcomes the problem of the knowledge of the exact likelihood calculation by means of simulations and a set of quantities, called “summary statistics” (SSs) that summarize the data.
Aim
The project of PhD thesis is aimed to a general improvement of such a method and to a wider application of it. In particular one would like to:
1) Extend to a genome wide scale the study, in particular with regard to genes involved in complex diseases in regions under selective pressure.
2) Improve the methods both in terms of computational efficiency and of quality of the estimations;
3) Generalize the applicability of the method to a wider range of scenarios (selection on standing variations, variants undergone selection but currently fixed, more complex demographic scenarios, regions with different recombination rates, and impact of epigenetic modifications, etc.);